Richard Gonzalez
Center Director, Research Center for Group Dynamics, Institute for Social Research
Director, BioSocial Methods Collaborative, RCGD
Amos N Tversky Collegiate Professor, Psychology and Statistics, LSA
Professor of Marketing, Stephen M Ross School of Business
Professor of Integrative Systems and Design, College of Engineering
E-mail: | Email Richard Gonzalez |
Address: | Research Center for Group Dynamics Institute for Social Research University of Michigan 426 Thompson Street Ann Arbor, Michigan 48106 |
Phone: | 734-647-6785 |
When multidimensional scaling goes random, random effects that is….
This paper expands individual-difference multidimensional scaling to include random effect terms. This technique can be used when each case produces a distance matrix. The model estimates a common space across units except for case-specific weight parameters that scale each dimension. That idea had been around for decades. Our contribution was to extend it by placing the model in the context of random effects, allowing one to build linear models for those case-specific weight parameters. It was an early paper using random effects on a large nonlinear problem. It was a lot of fun working on this technical paper with Doug Clarkson. He taught me a lot about modern computational algorithms in statistics, including MCEM and nonparametric approaches.
Clarkson, D. B. & Gonzalez, R. (2001). Random effects diagonal metric multidimensional scaling models. Psychometrika, 66, 25-43. doi:10.1007/BF02295730 (PDF)
Abstract
By assuming a distribution for the subject weights in a diagonal metric (INDSCAL) multidimensional scaling model, the subject weights become random effects. Including random effects in multidimensional scaling models offers several advantages over traditional diagonal metric models such as those fitted by the INDSCAL, ALSCAL, and other multidimensional scaling programs. Unlike traditional models, the number of parameters does not increase with the number of subjects, and, because the distribution of the subject weights is modeled, the construction of linear models of the subject weights and the testing of those models is immediate. Here we define a random effects diagonal metric multidimensional scaling model, give computational algorithms, describe our experiences with these algorithms, and provide an example illustrating the use of the model and algorithms.
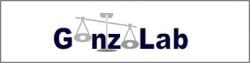